Blob Usage Strategies by Rollups and Non-rollup Applications
This report provides an in-depth analysis of blob-carrying type 3 transactions.
Jason @0xbbbb_eth
Account Abstraction Developer
MEV Researcher
Core Contributor of Panta Rhei
TDLR
The main applications using blobs are rollups, accounting for approximately 87%. Non-rollup applications mainly include Blobscriptions and customized type 3 transactions.
Rollup applications choose different blob usage strategies according to their own situations. The strategies will consider the number of blobs carried by type 3 transactions, blob utilization, and blob submission frequency to balance the costs of availability data fees and delay costs.
Non-rollup applications can be characterized and distinguished from rollup applications by the number of blobs carried by type 3 transactions, blob utilization, and blob submission frequency. These features help identify scenarios of blob abuse, allowing for the design of corresponding anti-abuse mechanisms.
In most cases, using blobs as a data availability solution is more cost-effective than calldata. However, there are a few scenarios where calldata is cheaper: blob gas price spikes and blob utilization is extremely low.
Short-term fluctuations in blob gas price is mainly influenced by the demand from non-rollup applications. Rollup applications have a relatively inelastic demand for blobs, so they do not significantly impact short-term fluctuations in blob gas prices.
Currently, rollup applications do not seem to consider blob gas price as a reference factor in their blob usage strategies.
The probability of blocks containing type 3 transactions being reorganized is extremely low. Additionally, carrying more blobs does not increase the probability of block reorganization. However, there is a clustering phenomenon in block height for blocks containing type 3 transactions.
Introduction
This report provides an in-depth analysis of type 3 transactions used for carrying blobs from the time of the Ethereum Decun upgrade until May 22, 2024. It focuses on blob usage strategies of rollup and non-rollup applications. The dataset, data processing programs, and visualization code for this report are open source, detailed in the following "Dataset" section.
For more information on the EIP-4844 fee mechanism and rollup data availability strategies, see the author's two articles:
EIP-4844 Economics #1: In-depth analysis of EIP-4844 fee mechanism: Chinese | English
EIP-4844 Economics #2: In-depth analysis of Rollup data availability strategies: Chinese | English
Type 3 Transactions & Blobs Share by Applications
This report analyzed 348,121 type 3 transactions included in the canonical blocks, and calculated the transaction and blob distribution across different applications, as shown in Figures 1 and 2.
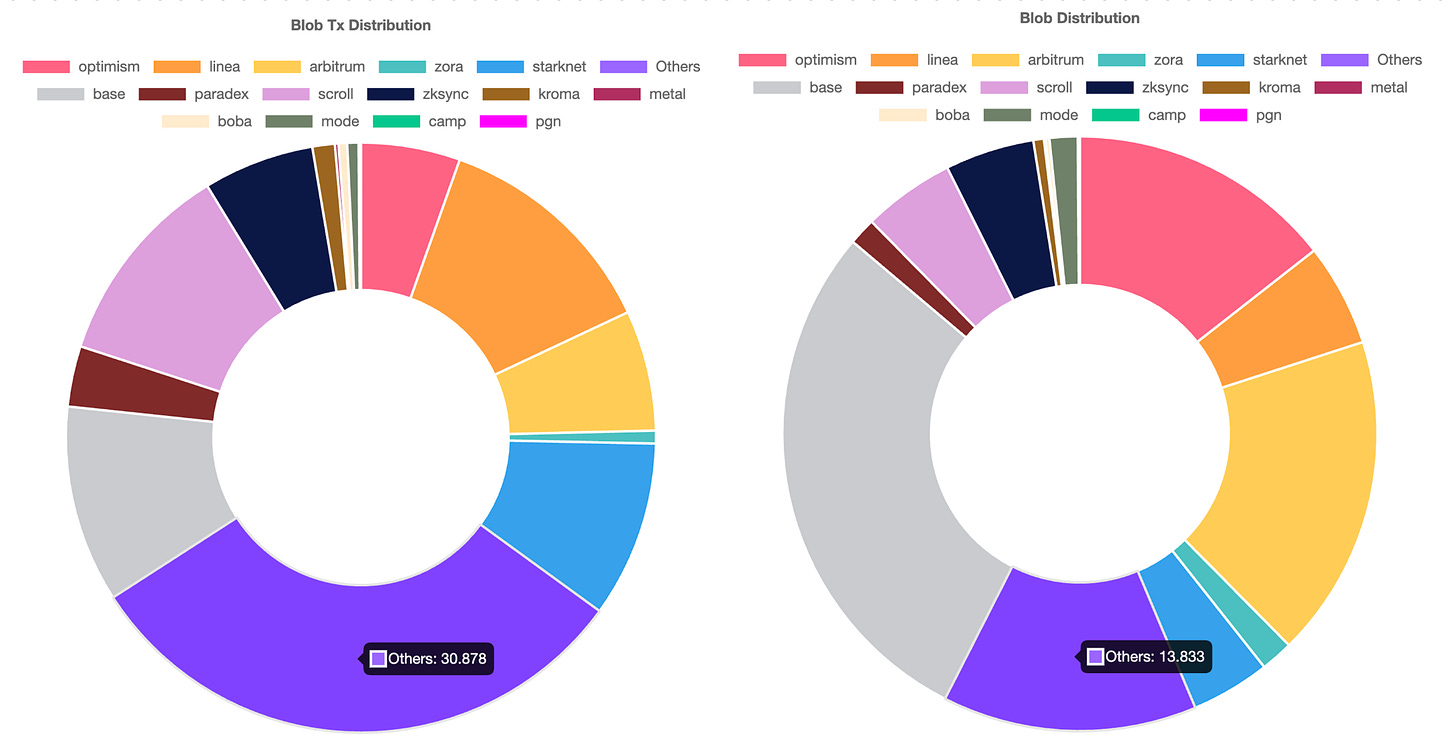
Due to the significant differences between Rollup applications and non-Rollup applications (labeled as Others in the figures), they are discussed separately.
Rollup Applications
Figures 1 and 2 show that approximately 69% of type 3 transactions and 86% of blobs are posted by rollups, indicating that EIP-4844 achieves its design goal: replacing calldata with blobs as the data availability solution for rollups.
Observations from Figure 1 on the proportion of type 3 transactions:
Base, Scroll, Linea, and Starknet are in the same tier, having the highest transaction proportions.
Arbitrum, Optimism, and Zksync are in the next tier, having the second-highest transaction proportions.
This phenomenon seems counterintuitive as Arbitrum and Optimism have higher TPS than Scroll, Linea, and Starknet and should have a higher proportion of type 3 transactions.
Figure 2 shows that counterintuitive phenomenon is caused by different rollup strategies in the number of blobs carried by type 3 transactions.
Observations from Figure 2 on the proportion of blobs:
Base stands alone, having the highest proportion of blobs.
Arbitrum and Optimism are in the same tier, having the second-highest proportion of blobs.
Scroll, Linea, Starknet, and Zksync are in the same tier, having a medium proportion of blobs.
This phenomenon aligns more with intuition: blob proportions are directly related to the scale of rollup's availability data, thus showing a positive correlation with rollup TPS.
💡 The real-time TPS of various Rollups can be seen here.
The differences in the proportions of various rollups in Figure 1 and Figure 2 indicate that rollups adopt different strategies regarding the number of blobs carried by type 3 transactions. For example, Arbitrum and Optimism's type 3 transactions carry more blobs compared to Scroll, Linea, and Starknet.
It is worth considering: why don't some rollups carry more blobs, given that more blobs can better amortize the fixed costs of type 3 transactions?
An important reason may be that rollups need to consider not only the fee costs of availability solutions but also the delay costs. Delay costs are implicit, mainly affecting user experience, the security model of some decentralized applications, and the liveness of some decentralized applications.
For high TPS rollups like Base, Arbitrum, and Optimism, the availability data can fill up to 6 blobs within a short period, reaching the block limit. These rollups can pursue the lowest fee costs while having low delay costs.
For medium TPS rollups like Scroll, Linea, Starknet, and Zksync, the availability data cannot fill up to 6 blobs within a short period. These rollups cannot pursue the lowest fee costs to maintain acceptable delay costs, meaning their type 3 transactions can only carry fewer than 6 blobs.
💡 Another important reason may be that the validity proof or fraud proof mechanisms of some rollups cannot handle multi-blob scenarios currently.
Non-Rollup Applications
Besides the expected rollup applications, there are actually many non-rollup applications. Figures 1 and 2 show that about 31% of type 3 transactions and 14% of blobs are posted by non-rollup applications (labeled as Others in the figures).
Typical non-rollup applications are as follows:
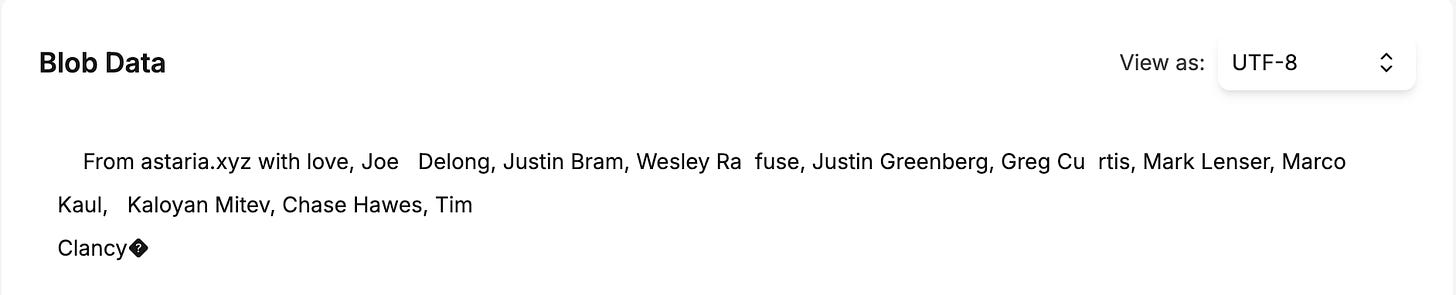
The difference between the proportion of type 3 transactions (31%) and blobs (14%) for non-rollup applications indicates that non-rollup applications and rollup applications have different needs.
Rollup applications are B2B businesses aiming to fill fine-grained Layer 2 transaction availability data, so their type 3 transactions are not limited to carrying only 1 blob.
Non-rollup applications are B2C businesses aiming to upload complete text, images, etc., so their type 3 transactions usually carry only 1 blob to meet their needs.
In-depth Analysis of Rollup Blob Usage Strategies
The preliminary analysis above shows that different rollups have made complex trade-offs in their blob usage strategies. This section models the rollup blob usage strategies and empirically analyzes different rollup strategies based on real rollup data (the number of blobs carried by type 3 transactions, blob utilization, and blob submission intervals).
Rollup Strategy Model
Given the tps
of a rollup and its blob submission interval blobInterval
, the availability data scale submitted by the rollup can be obtained (1) :
k1
: constant
Given the number of blobs carried by type 3 transactions blobNumber
and the blob utilization blobUtilization
(the proportion of non-empty bytes in the blob), the availability data scale submitted by the rollup can also be obtained (2) :
k2
: constant
Combining equations (1) and (2), we get (3) :
k
: constant
The fee cost per transaction for rollups is expressed as (4) :
fixedCost
: the fixed cost of a type 3 transactionblobCost
: the cost of a single blob
Combining equations (3) and (4), we get (5) :
The delay cost per transaction for rollups is expressed as (6) :
F
: monotonic increasing functionThe larger the
blobInterval
, the larger the delay costdelayCost
per transaction.
Combining equations (3) and (6), we get (7) :
The larger the
blobUtilization
, the larger the delay costdelayCost
.The larger the
blobNumber
, the larger the delay costdelayCost
.The larger the
tps
, the smaller the delay costdelayCost
.
Empirical Analysis 1: Distribution of Blobs Carried by Type 3 Transactions (blobNumber
)
Based on the rollup strategy model, it is evident that rollups make trade-offs in choosing the number of blobs carried by type 3 transactions:
According to equation (5), carrying more blobs in type 3 transactions means lower fee costs.
According to equation (7), carrying more blobs in type 3 transactions means lower delay costs.
The report summarizes the distribution of the number of blobs carried by type 3 transactions by rollups (see Figure 5) to analyze the strategy preferences of different rollups.
Figure 5 shows that most rollups tend to carry a fixed number of blobs in type 3 transactions:
Empirical Analysis 2: Distribution of Blob Utilization (blobUtilization
)
Based on the rollup strategy model, rollups also make trade-offs in blob utilization:
According to equation (5), higher blob utilization means lower fee costs.
Compared to increasing the number of blobs carried by type 3 transactions, increasing blob utilization reduces fees more effectively because it impacts both the amortized cost of fixed fees and blob fees.
According to equation (7), higher blob utilization means lower fee costs.
The report summarizes the distribution of blob utilization by rollups (see Figure 6) to analyze the strategy preferences of different rollups.
Figure 6 shows that the blob utilization for all rollup applications is above 20%. Additionally, different rollups have different preferences for blob utilization:
Empirical Analysis 3: Distribution of Blob Submission Intervals (blobInterval
)
According to equation (5), fee costs can be controlled by rollups actively adjusting the number of blobs carried by type 3 transactions (blobNumber
) and blob utilization (blobUtilization
).
According to equation (7), delay costs can be controlled by rollups actively adjusting the number of blobs carried by type 3 transactions (blobNumber
) and blob utilization (blobUtilization
) based on their TPS.
The report summarizes the blob submission intervals for rollups (see Figure 7) to analyze the delay costs of different rollup strategies.
Figure 7 shows that different rollups have different preferences for delay costs:
Rollup Strategy Analysis
Combining the above empirical analyses, the rollup strategies can be categorized as follows:
In-depth Analysis of Non-Rollup Blob Strategies
Rollup applications are B2B, while non-rollup applications are B2C. Therefore, non-rollup applications differ from the rollup strategy model. For non-rollup applications:
The number of blobs carried by type 3 transactions depends on the size of the content (texts/images) stored in the blobs.
Blob utilization depends on the size of the content (texts/images) stored in the blobs.
Blob submission intervals depend on the immediate needs of C-end users, with no delay costs involved.
According to Figure 5 (Others), 1 blob can meet the needs of most non-rollup applications.
According to Figure 6 (Others), the blob utilization is concentrated between 20% and 40%, indicating that non-rollup applications generally cannot fill the blob, with the data size mainly between 25.6 kB and 51.2 kB.
According to Figure 7 (Others), about 83% of blobs have a submission interval of less than 1 minute, indicating a relative high frequency of user demand for non-rollup applications.
In summary, the type 3 transactions for non-rollup applications can be characterized as: high-frequency transactions carrying 1 low-utilization blob. The essence of this characterization is that non-rollup applications are driven by immediate needs and are less concerned about the fee cost per data byte compared to rollup applications. This characterization allows for the identification of non-rollup applications, which in turn helps design mechanisms to limit blob abuse by non-rollup applications.
Is Using Blobs Always More Cost-effective than Calldata?
Before EIP-4844, applications mainly used calldata as a data availability solution. After EIP-4844, applications switched to using blobs. However, is using blobs always more cost-effective than calldata? In fact, for some applications, the container pricing model of blobs can be more expensive than the pay-as-you-go model of calldata in certain scenarios.
Introducing feeRatio
to measure the relative advantages of the two solutions (8) :
When
feeRatio
≥ 1, it indicates that using blobs as a data availability solution is not worse than calldata.When
feeRatio
< 1, it indicates that using blobs as a data availability solution is worse than calldata.
The report summarizes the feeRatio
for each type 3 transaction, as shown in Figure 8:
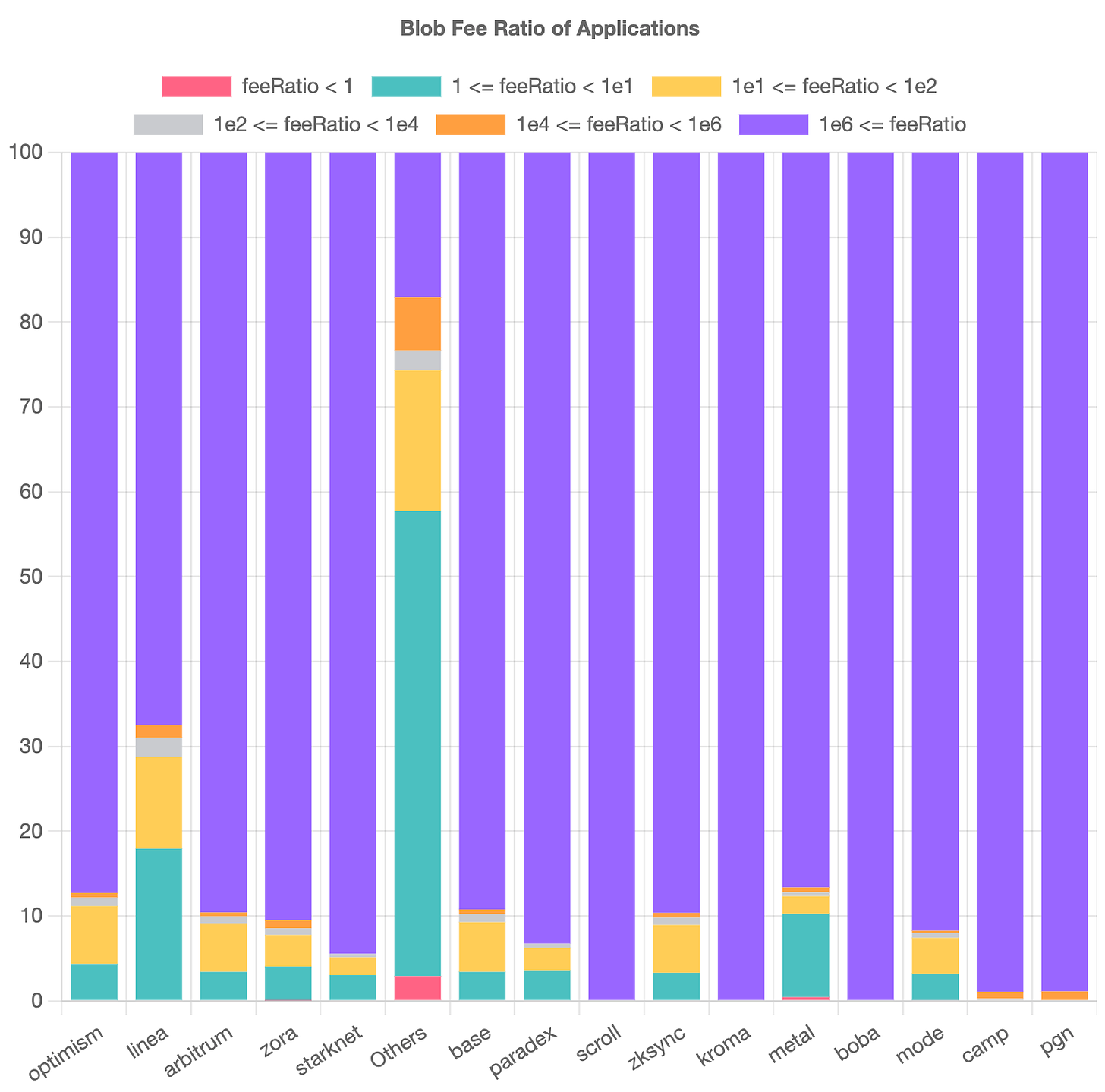
Figure 8 shows that in most cases, blobs are more cost-effective than calldata, with feeRatio
> 1. Additionally, the actual effect is astonishing, with feeRatio
mainly in the range of ≥ 1e6
, reducing costs by a factor of 1 million. This impressive effect is mainly due to the blobBaseFee
being long-term stable at 1.
Figure 8 also shows a few cases where feeRatio
< 1 (red), indicating that calldata is more cost-effective than blobs:
Mostly in non-Rollup applications (Others):
Non-rollup applications generally do not care about the cost differences between blobs and calldata; they care about using blobs itself, such as in Blobscriptions.
A few in Metal rollup:
Rollup application Metal seems not to have considered switching between blobs and calldata in its strategy, leading to suboptimal choices in some extreme cases.
Extreme cases are mainly due to Metal's low blob utilization (see Figure 6) coinciding with a spike in blob gas prices.
However, given that extreme scenarios are rare and maintaining two data availability solutions is costly, Metal's suboptimal strategy in extreme cases seems acceptable.
💡 The analysis of blob and calldata solutions in this section only considers fee costs and not delay costs. Considering delay costs, calldata has an actual advantage.
Blob Gas Price and Blob Usage Strategies
Analysis of Blob Gas Price Fluctuations
The report summarizes the proportion of type 3 transactions and blobs from different applications under various blob gas prices:
Figures 9 and 10 show that in scenarios of high blob gas prices (> 10), the proportion of non-rollup applications (Others) is significantly higher than in scenarios of low blob gas prices (< 10).
Therefore, it can be concluded that the surge in blob gas prices is mainly driven by the demand from non-rollup applications, rather than rollup applications. Otherwise, the proportion of rollup and non-rollup applications should remain stable.
How Rollups Respond to Blob Gas Price Fluctuations
Additionally, in Figures 9 and 10, readers might notice that some rollup applications seem to respond to high blob gas prices.
For example, Scroll has a higher proportion under low blob gas prices (< 10) but appears to have a proportion of 0 under high blob gas prices (> 10). Scroll seems to suspend blob submissions under high blob gas prices.
However, this conclusion is incorrect. The reason is that not all rollups immediately used blobs after the EIP-4844 upgrade.
The report summarizes the block height distribution of type 3 transactions for different applications (see Figure 11), revealing that Scroll's proportion of 0 under high blob gas prices (> 10) is mainly because it started using blobs after the high blob gas price period.
By retaining only the rollups that used blobs immediately after the EIP-4844 upgrade (see Figures 12 and 13), the proportion of type 3 transactions and blobs for these rollups can be observed under different blob gas prices to understand how rollups respond to blob gas prices.
Figures 12 and 13 show that the proportion of various rollups remains stable under different blob gas prices. Rollups do not seem sensitive to blob gas prices, likely due to the rigid demand for availability data by rollups.
💡 Another possibility is that all rollups reduce the frequency of blob submissions under high blob gas prices. Since all rollups must move in sync to maintain stable proportions, this scenario is unlikely.
Number of Blobs Carried by Type 3 Transactions under Different Blob Gas Prices
Hypothesis: The higher the blob gas price, to reduce fee costs, applications should carry more blobs in type 3 transactions, i.e., the number of blobs should be positively correlated with blob gas prices.
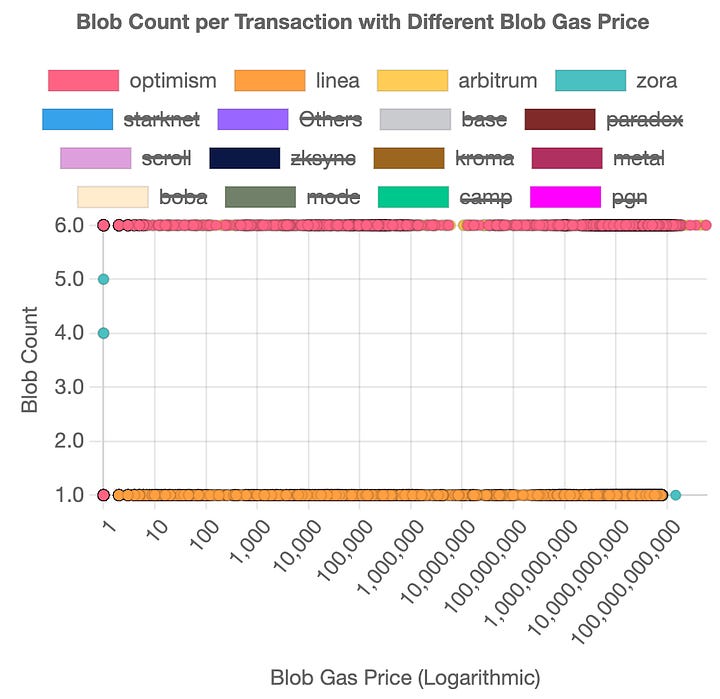
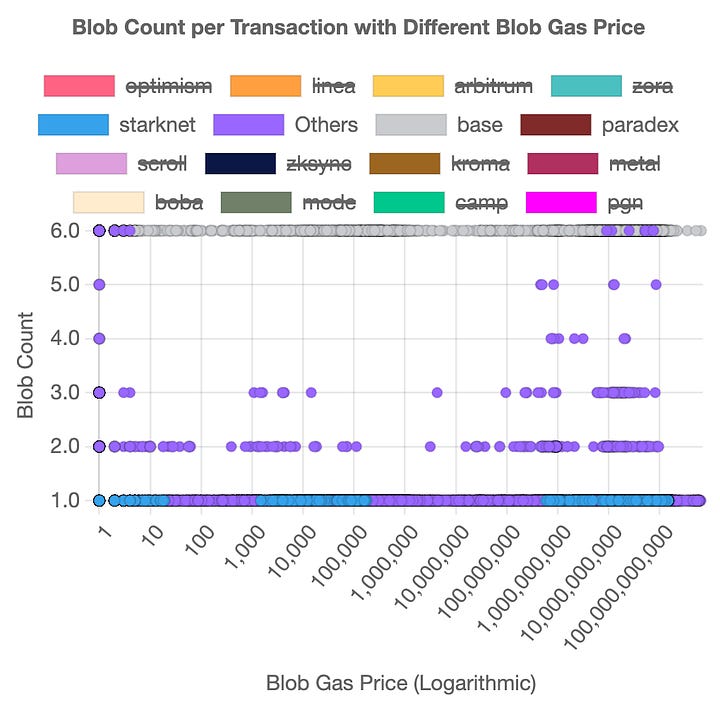
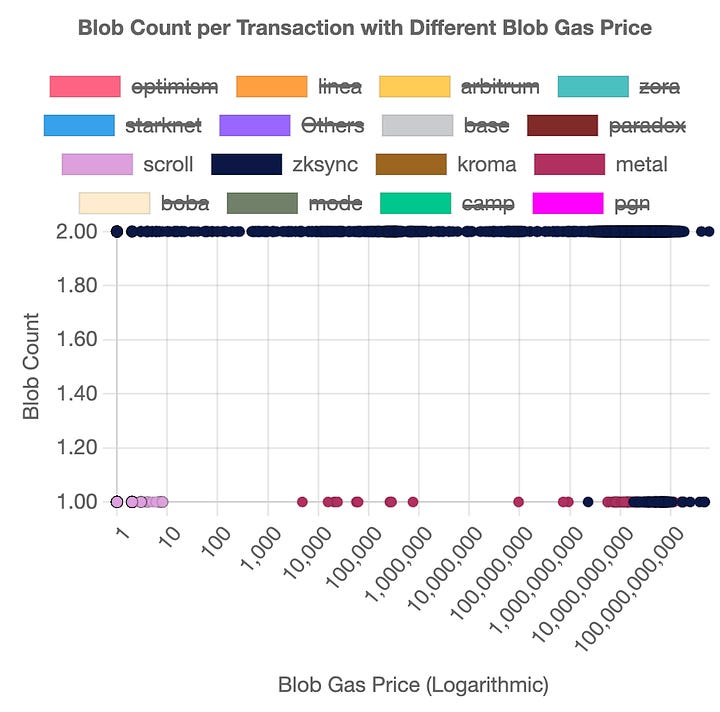
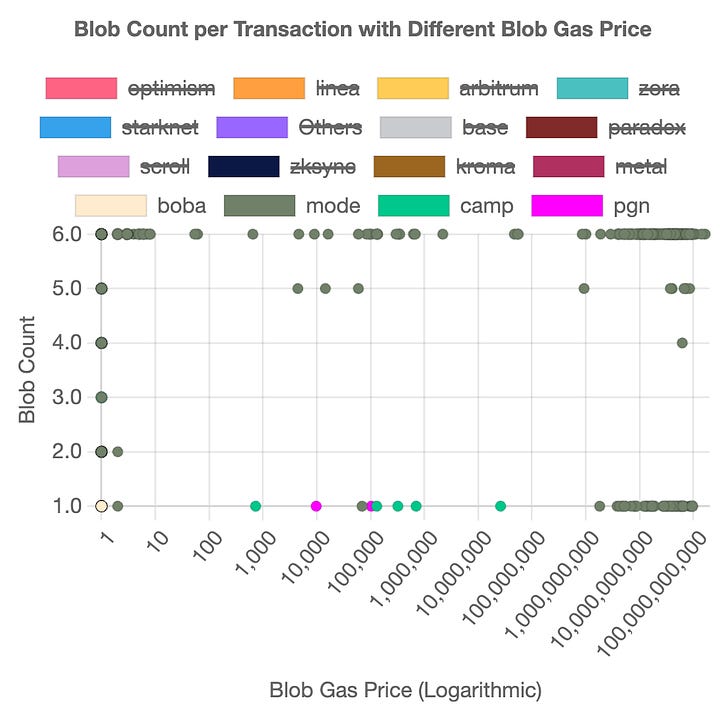
Figure 14 shows that the hypothesis does not hold. The number of blobs carried by type 3 transactions is unrelated to blob gas prices, indicating that current applications do not consider blob gas prices when choosing the number of blobs carried by type 3 transactions.
Blob Utilization under Different Blob Gas Prices
Hypothesis: The higher the blob gas price, to reduce fee costs, applications should increase blob utilization, i.e., blob utilization should be positively correlated with blob gas prices.
Figure 15 shows that the hypothesis does not hold. Blob utilization is unrelated to blob gas prices, indicating that current applications do not consider blob gas prices when choosing blob utilization.
Blob Submission Intervals under Different Blob Gas Prices
Hypothesis: The higher the blob gas price, to reduce fee costs, applications should delay blob submissions, i.e., blob submission intervals should be positively correlated with blob gas prices.
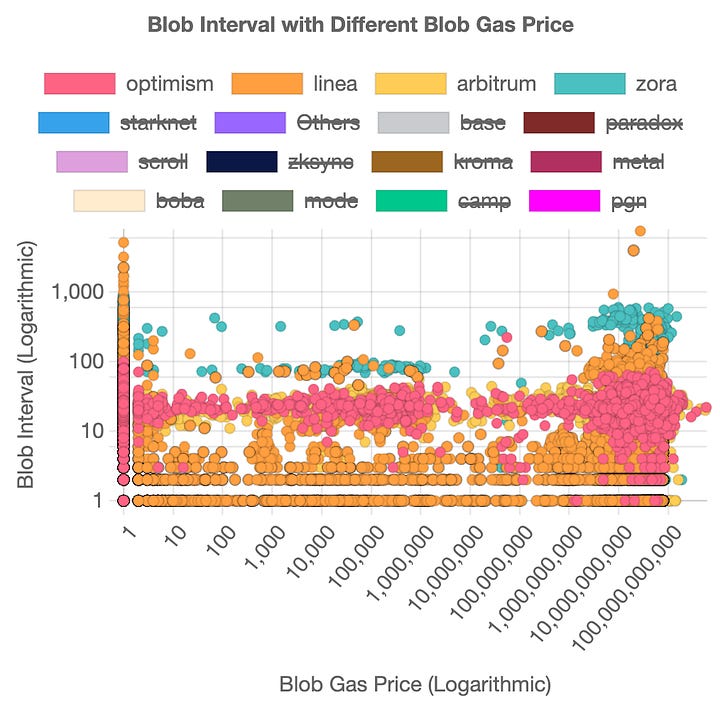
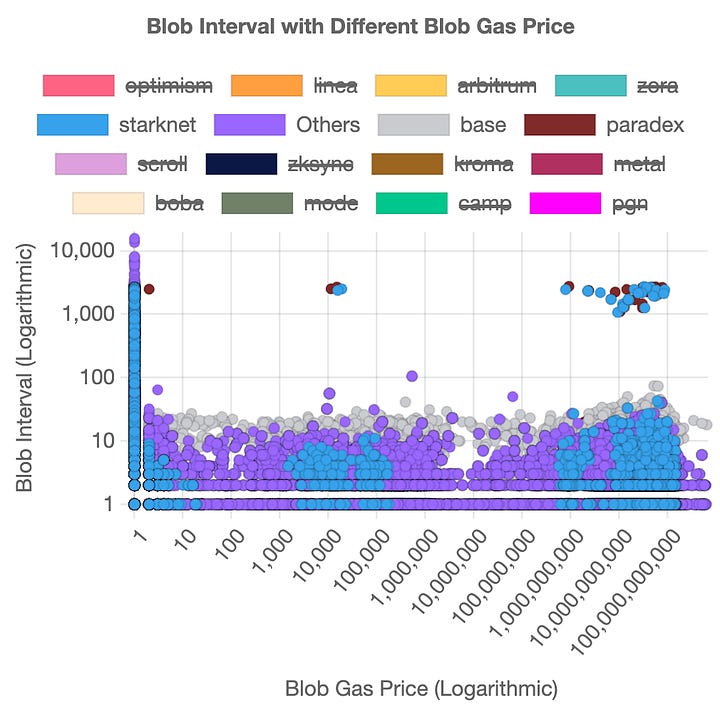
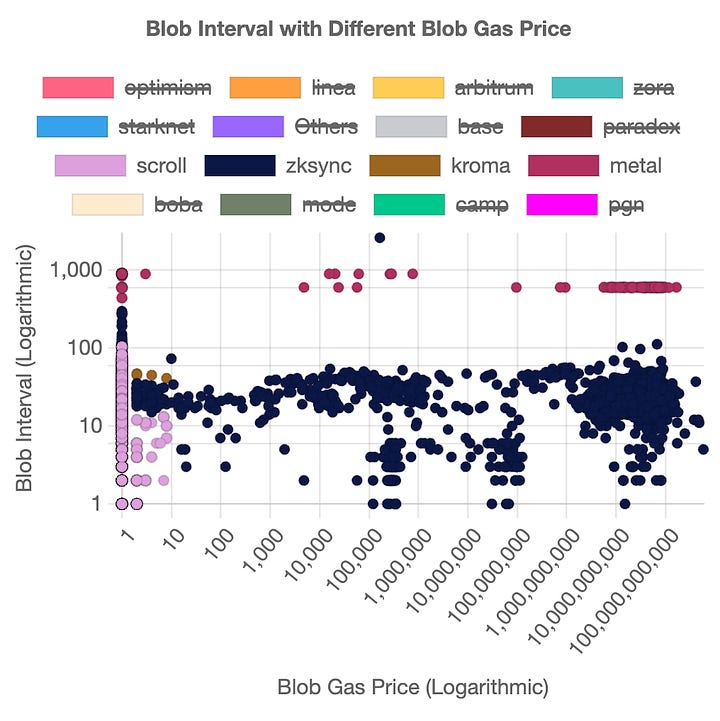
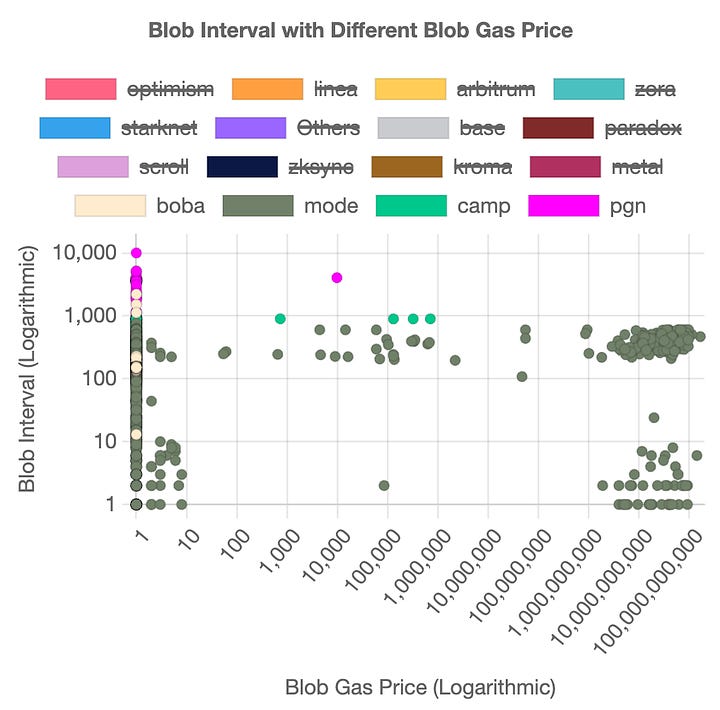
Figure 16 shows that the hypothesis does not hold. Blob submission intervals are unrelated to blob gas prices, indicating that current applications do not consider blob gas prices when choosing blob submission intervals.
Blobs and Block Reorg
According to statistics, from the Decun upgrade to May 22, there were 171 type 3 transactions included in the forked blocks and 348,121 included in the canonical blocks. Therefore, the proportion of type 3 transactions being forked is approximately 0.049% (= 171 / (171+348121)). This section explores the relationship between block reorg and blob.
Blob Number Distribution in the Canonical and Forked Blocks with Blobs
The report summarizes the distribution of blobs in the canonical and forked blocks (see Figure 17) to try to derive some information from the differences.
Hypothesis: More blobs increase the probability of block reorganizations.
If the hypothesis holds, the following inequality should be satisfied (9):
According to Bayes' theorem, equation (9) is equivalent to (10):
We check whether the actual data satisfies equation (10) and obtain the following table:
The table above shows that equation (10) does not hold for all n
. Therefore, the hypothesis does not hold, indicating that more blobs are not significantly related to block reorganizations.
💡 Since the proportion of type 3 transactions included in forked blocks is extremely low,
P(blob=n)
can be approximated byP(blob=n|normal)
, wherenormal
refers to inclusion in the canonical blocks.
Distribution of Type 3 Transactions and Blobs by Applications in the Canonical and Forked Blocks with Blobs
The report summarizes the distribution of type 3 transactions and blobs by application in the canonical and forked blocks (see Figures 18 and 19) to try to derive some information from the differences.
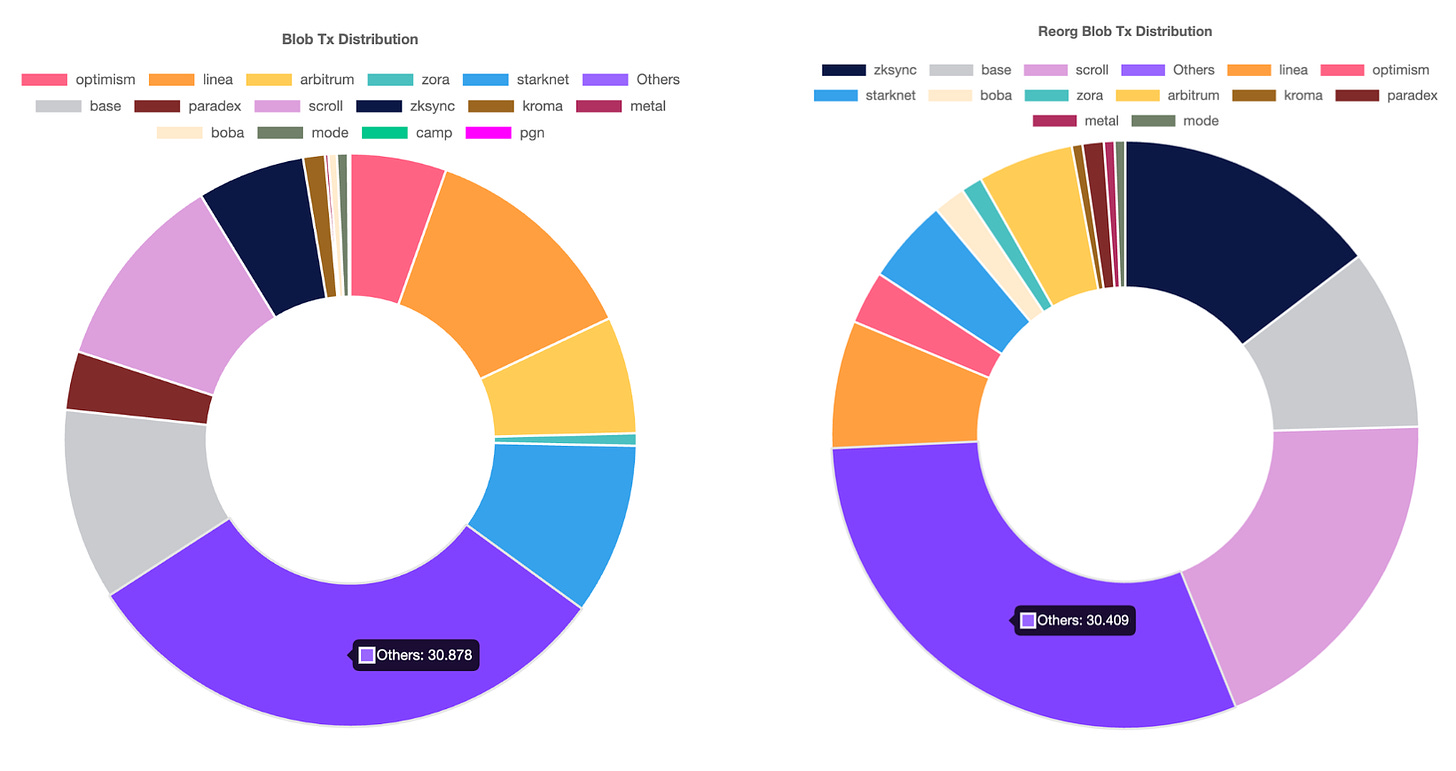
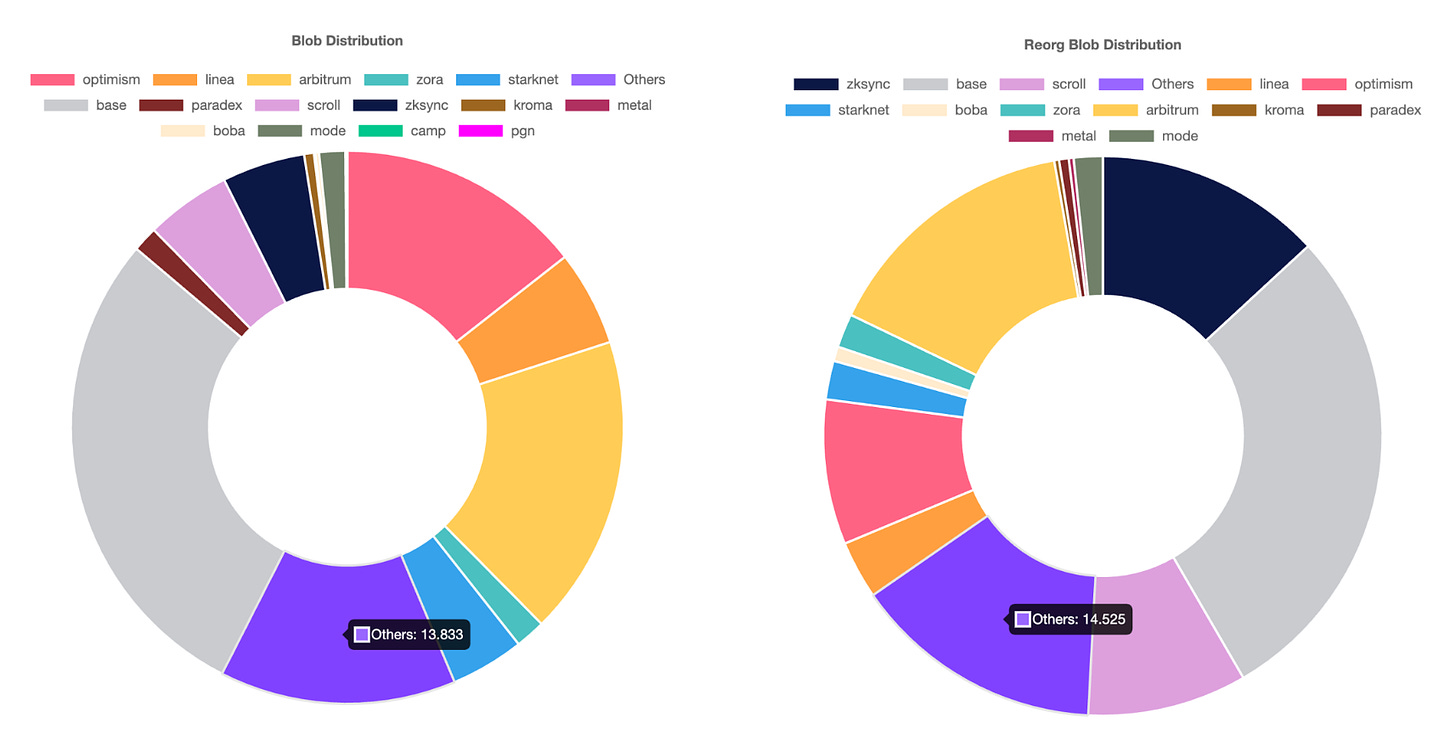
Hypothesis: Applications are unrelated to block reorganizations. Therefore, the application distribution for reorganized blocks should be similar to the distribution for the canonical blocks.
Figures 18 and 19 show that the proportion of type 3 transactions/blobs for Zksync and Scroll in forked blocks is significantly higher than in the canonical blocks, indicating that the hypothesis does not hold.
Equation (11) and (12)
Applications seem to have some connection with block reorganizations, possibly related to differences in blob usage strategies by applications:
Zksync and Scroll are less strategic in selecting the timing of submitting type 3 transactions, targeting block heights prone to reorganization.
The unique characteristics of Zksync and Scroll's type 3 transactions make the blocks containing them more likely to be reorganized.
Clustering Phenomenon of Forked Blocks with Blobs
The report summarizes the distribution of type 3 transactions by application in the canonical and forked blocks (see Figure 20) to try to derive some information from the differences.
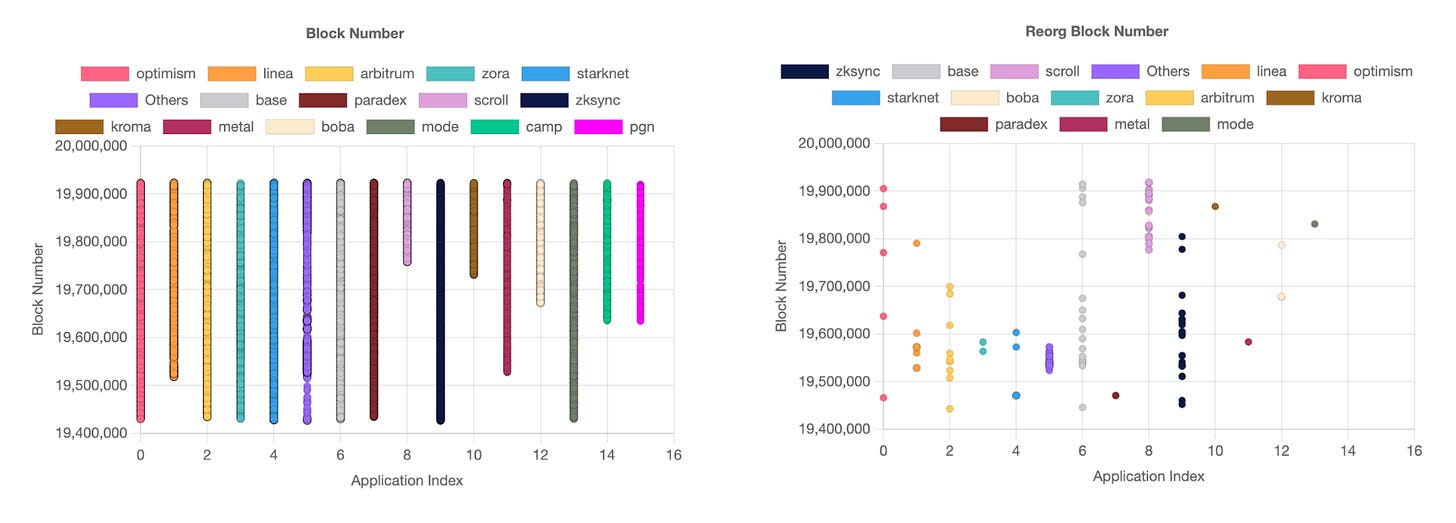
If each block has the same probability of being reorganized, the forked blocks should be evenly distributed across the block height range. However, Figure 20 shows a clustering phenomenon in block heights for forked blocks, possibly related to network conditions.
In addition, the clustering phenomenon that occurs in block reorganization seems to be somewhat related to the applications that submit blobs. For example, type 3 transactions for non rollup applications are only included in forked blocks between 19500000 and 19600000.
Dataset
This report collected data on type 3 transactions with block heights between 19426589 and 19922944 from blobscan, including 348,121 type 3 transactions included in the canonical blocks and 171 included in forked blocks.
💡 The Decun upgrade activation height is 19426589, corresponding to Mar-13-2024 01:55:59 PM +UTC to May-22-2024 04:07:23 AM +UTC for block heights between 19426589 and 19922944.
Data source: blobscan
Data processing script: GitHub repository
Processed data: GitHub repository
Data statistics and visualization: GitHub repository
Basic Data Used in the Report
The data used in the report is processed through a custom script, resulting in:
{
"hash" : "0x000001bc628a7415eff93e5cb296b0f02a163e24e60453dce163f18113ada54f",
"blockNumber" : 19850392,
"blockHash" : "0x6b6e089b6cb192b38b637592d5eda052e38c0b187df4f25d5119cae3d5c965ae",
"blobCount" : 6,
"rollup" : "optimism",
"blobGasPrice" : 1,
"blobInterval" : 22,
"gasRatio" : 15.559494018554688,
"feeRatio" : 80990325270.61229,
}
hash
: type 3 transaction hashblobNumber
: block height of the type 3 transactionblockHash
: block hash of the type 3 transactionblobCount
: number of blobs carried by the type 3 transactionrollup
: initiating application of the type 3 transaction, including rollup and non-rollup applications (marked as Others)blobGasPrice
: blob gas price paid by the type 3 transaction for blob data gasblobInterval
: block distance from the last type 3 transaction by this application. Based on an interval of 12 seconds per slot, the time interval of application submissions can be approximated (note: precise calculation requires considering missed slots).gasRatio
: ratio of gas used when converted to calldata compared to current blob data gas usage, i.e.,blobAsCalldataGasUsed / blobGasUsed
.gasRatio
can approximate blob utilization.feeRatio
: ratio of calldata fees to current blob fees, i.e.,blobAsCalldataFee / blobFee
If
feeRatio
< 1, calldata is more cost-effective than blobs, yet the transaction chose blobs.If
feeRatio
> 1, blobs are more cost-effective than calldata, and the transaction chose blobs.
Calculating Blob Utilization
Blob utilization blobUtilization
can be cleverly derived based on gasRatio
.
Equations (13), (14), (15), and (16):
Combining equations (13), (14), (15), and (16), we get (17):
Where k
represents the proportion of non-zero bytes in availability data.
For specific applications,
k
is generally stable.Generally, the proportion of zero bytes is much lower than that of non-zero bytes, and
k
can be approximated as 1.
Summary
The strategies for using blobs are personalized and complex. This report attempts to abstract, normalize and empirically analyze these strategies.
For rollup applications, it is especially important to balance fee costs and delay costs by adopting appropriate strategies based on their own and Ethereum network conditions.
For non-rollup applications, it is crucial to identify scenarios of blob abuse to ensure the sustainability of the blob ecosystem.
For the protocol layer, analyzing how applications use type 3 transactions provides valuable insights into the impact of blobs on the protocol layer, such as block reorganizations, better blob fee mechanisms, and mechanisms to prevent blob abuse.